Soil moisture from EUMETSAT ASCAT
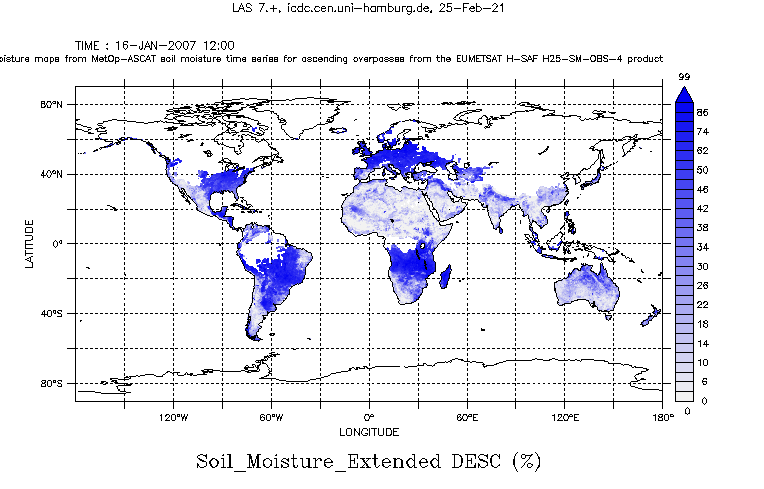
Topics
- Access
- Description
- Parameters
- Coverage, spatial and temporal resolution
- Data quality
- Contact person
- References
- Data citation
Access
UNRESTRICTED:
- View monthly soil moisture data at LAS
- Get monthly soil moisture data via THREDDS (get all with wget script)
- Access monthly soil moisture data via OPeNDAP
- Access daily or monthly soil moisture data via the ZFDM repository
RESTRICTED: This link to the data set is only available for a restricted user group. The data set is only accessible in CEN/MPI net or accessible from external nets with a customer account. Please contact ICDC if you would like to access this data from outside the network.
- View daily soil moisture data at LAS
- Access daily soil moisture data via OPeNDAP
- Data access via file system: /data/icdc/land/ascat_soilmoisture
Description
This soil moisture data set is based on radar backscatter measurements (at C-Band) of the Advanced SCATterometer (ASCAT) aboard the EUMETSAT MetOp satellite (see references). This data is first normalized to a common incidence angle (40°) using a radar backscatter model. The obtained radar backscatter coefficient is a function of the soil moisture: low values correspond to a low soil moisture, high values are associated with a high soil moisture. Radar backscatter values are scaled between 0 % (dry soil) and 100% (wet soil, saturated with water). The obtained relative soil moisture values represent the moisture in the topmost 2-5 cm of the soil.
The offfered data sets are based on the reprocessed version v7 of this EUMETSAT H-SAF product: ASCAT SSM CDR v7 12.5 km: H119 and ASCAT SSM CDR v7 EXT 12.5 km: H120. H120 is the offline extension of the H119 soil moisture CDR, i.e. the interim CDR (iCDR). The original data of this product are organized as time-series of the soil moisture per grid cell.
We offer daily and monthly global maps of the 5-day composite / mean soil moisture computed separately for ascending and descending satellite overpasses and projected onto a simple cartesian coordinate grid with about 0.1° x 0.1° grid resolution. The separation into ascending and descending data is motivated by work such as done by Al-Yaari, A., et al. (see references).
The Algorithm Theoretical Basis Document (ATBD) for H119 and H120 products is given in the references.
The original data, i.e. the soil moisture time series, can be retrieved from EUMETSAT H-SAF (see references).
Last update of data set at ICDC: August 3, 2023.
Parameters
Name | Unit/Range | Comment |
---|---|---|
relative soil moisture [variable "sm"] | 0 ... 100% | based on correction flag 0 = best quality (see Product User Guide) |
relative error / noise [variable "sm_noise"] | 0 ... 100% | for the parameter above |
relative soil moisture extended [variable "sm_ext"] | 0 ... 100% | based on Processing Flags 0, 1, 2, and 16 (see Product User Guide) |
relative error / noise extended [variable "sm_ext_noise"] | 0 ... 100% | for the parameter above |
status flag | 0 ... 127 | Combination of surface state flag, correction flag and processing flag (see Product User Guide and description in netCDF file) |
N | 0 ... 5 | Number of overpasses per grid cell within 5-day interval |
snow cover | 0 ... 100% | climatology, based on SSM/I data set |
frozen soil | 0 ... 100% | climatology, based on ERA40 data |
wetlands / lakes / rivers | 0 ... 100% | static, based on GLWD (2004) and GSHHS (v1.5, 2004) data |
complexity of topography | 0 ... 100% | static, based on GTOPO30 data |
soil porosity (LDAS) | 0 ... 1 m³ / m³ | re-sampled from Land Data Assimilation System (LDAS) data (NASA, Reynolds, 2000) |
soil porosity (HWSD) | 0 ... 1 m³ / m³ | computed from HWSD by applying the formulas of Saxton and Rawls (2006) |
Name | Unit/Range | Comment |
---|---|---|
relative soil moisture extended | 0 ... 100% | derived from daily variable "sm_ext" |
relative error / noise extended | 0 ... 100% | derived from daily variable "sm_ext_noise" |
N_days [variable "n_of_days"] | 0 ... 31 | number of days with valid data |
N [variable "n_of_overpasses"] | 0 ... 5 | mean number of overpasses per grid cell within 5-day interval |
snow cover | 0 ... 100% | climatology, based on SSM/I data set |
frozen soil | 0 ... 100% | climatology, based on ERA40 data |
wetlands / lakes / rivers | 0 ... 100% | static, based on GLWD (2004) and GSHHS (v1.5, 2004) data |
complexity of topography | 0 ... 100% | static, based on GTOPO30 data |
soil porosity (LDAS) | 0 ... 1 m³ / m³ | re-sampled from Land Data Assimilation System (LDAS) data (NASA, Reynolds, 2000) |
soil porosity (HWSD) | 0 ... 1 m³ / m³ | computed from HWSD by applying the formulas of Saxton and Rawls (2006) |
status flag | 0, 1, 2, 4, 8, 16, 32, 64, 127 | derived and simplified from daily status flag |
Coverage, spatial and temporal resolution
Period and temporal resolution:
- 2007-01-01 to 2022-12-31 (H119 until 2020-12-31, H120 afterwards)
- daily as sliding 5-day composites, separately for descending and ascending satellite overpasses
- 2007-01 to 2021-12 (H119 until 2020-12, H120 afterwards)
- monthly
Coverage and spatial resolution:
- Global
- Spatial resolution: about 0.1° x 0.1°, cartesian grid
- Geographic longitude: 179.944°W to 179.944°E
- Geographic latitude: 89.9437°S to 89.9437°N
- Dimension: 1599 rows x 3207 columns
- Altitude: following terrain
Format:
- NetCDF
Data quality
This data set contains a number of quality flags and additional information. One is the retrieval noise. This is estimated using Gaussian error propagation of the input uncertainties like the variability of the used radar backscatter values due to measurement noise and the variability of the sensitivity of the radar backscatter values to soil moisture for different soil and vegetation types.
We note, that the soil moisture retrieval method used here, is of limited use particularly in polar regions and regions covered by dense rain forest like in South America. These areas are therefore often flagged as unreliable data and/or show a large retrieval noise.
Soil moisture retrieval is not possible for areas covered with snow and ice, for areas with frozen soil and for wetlands/lakes/rivers. In order to allow identification of dubious soil moisture values (which have perhaps not been flagged as being unreliable), the product contains the percentage fractions of snow (variable: "snow_cover"), frozen soil (variable: "frozen_soil"), and wetlands (variable: "wetland") for every grid cell. Those for wetland are static, assuming that the fraction does not change over time, while those of snow and frozen soil stem from a climatology and therefore vary with season but don't have interannual variation.
Regions of a strongly variable topography are also problematic because of the highly variable local incidence angle in these cases. This causes problems to normalize the measured radar backscatter values to the common incidence angle and thus to retrieve the soil moisture. Therefore, the data set contains the normalized standard deviation of the altitude in each grid cell (in relative units) as a measure of the topographic complexity (variable: "topography").
A so-called "wet correction" is applied for dry regions classified as climate BW(h or k) according to Köppen; this is driven by the observation, that the time-series approach used to derive the soil moisture fails to provide realistic soil moisture values in those regions / cases where within the time period considered the soil moisture never reached 100%. Application of this wet correction and of regions where the sensitivity of the radar backscatter to the soil moisture is below 1% or where the soil moisture error is larger than 50% can be tracked in the quality flag.
Please see references for details.
Contact
Wolfgang Wagner
Department of Geodesy and Geoinformation (GEO), Research Group Remote Sensing
http://rs.geo.tuwien.ac.at, Vienna University of Technology (TU Wien)
email: ww (at) ipf.tuwien.ac.at or radar (at) ifp.tuwien.ac.atEUMETSAT
Hydrology - Satellite Application Facility (H-SAF)
http://hsaf.meteoam.it
email: us_hsaf (at) meteoam.itStefan Kern
ICDC / CEN / University of Hamburg
email: stefan.kern (at) uni-hamburg.de
References
Literature:
- Product User Manual (PUM) (pdf, not barrier free)
- Product Validation Report (PVR) (pdf, not barrier free)
- Algorithm Theoretical Basis Document (ATBD) EUMETSAT H SAF ATBD, ASCAT SSM CDR v5 12.5 km (H115), ASCAT SSM CDR v5 EXT 12.5 km (H116) (pdf, not barrier free)
- Bartalis, Z., Wagner, W., Naeimi, V., Hasenauer, S., Scipal, K., Bonekamp, H., Figa, J., and Anderson, C. (2007), Initial soil moisture retrievals from the METOP‐A Advanced Scatterometer (ASCAT), Geophys. Res. Lett., 34, L20401, doi:10.1029/2007GL031088. https://doi.org/10.1029/2007GL031088
- A. Al-Yaari, J.-P. Wigneron, A. Ducharne, Y.H. Kerr, W. Wagner, G. De Lannoy, R. Reichle, A. Al Bitar, W. Dorigo, P. Richaume, A. Mialon, (2014), Global-scale comparison of passive (SMOS) and active (ASCAT) satellite based microwave soil moisture retrievals with soil moisture simulations (MERRA-Land), Remote Sensing of Environment, 152, 614-626, doi:10.1016/j.rse.2014.07.013, https://doi.org/10.1016/j.rse.2014.07.013
- Jiangyuan Zeng, Zhen Li, Quan Chen, Haiyun Bi, Jianxiu Qiu, Pengfei Zou, (2015), Evaluation of remotely sensed and reanalysis soil moisture products over the Tibetan Plateau using in-situ observations, Remote Sensing of Environment, 163, 91-110, doi:10.1016/j.rse.2015.03.008, https://doi.org/10.1016/j.rse.2015.03.008
- C.M Holgate, R.A.M. De Jeu, A.I.J.M van Dijk, Y.Y Liu, L.J. Renzullo, Vinodkumar, I. Dharssi, R.M. Parinussa, R. Van Der Schalie, A. Gevaert, J. Walker, D. McJannet, J. Cleverly, V. Haverd, C.M. Trudinger, P.R. Briggs, 2016, Comparison of remotely sensed and modelled soil moisture data sets across Australia. Rem. Sens. Environ., 186, 479-500. doi:10.1016/j.rse.2016.09.015, https://doi.org/10.1016/j.rse.2016.09.015
- Hyunglok Kim, Robert Parinussa, Alexandra G. Konings, Wolfgang Wagner, Michael H. Cosh, Venkat Lakshmi, Muhammad Zohaib, Minha Choi,, Global-scale assessment and combination of SMAP with ASCAT (active) and AMSR2 (passive) soil moisture products (2018). Remote Sensing of Environment, 204, 260-275. doi:10.1016/j.rse.2017.10.026 , https://doi.org/10.1016/j.rse.2017.10.026
- Wagner, W., G. Lemoine, and H. Rott (1999), A method for estimating soil moisture from ERS scatterometer and soil data. Remote Sensing of Environment, 70(2), 191-207, doi:10.1016/S0034-4257(99)00036-X
- Naeimi, V., K. Scipal, Z. Bartalis, S. Hasenauer, and W. Wagner, 2009, An improved soil moisture retrieval algorithm for ERS and MetOp scatterometer observations, IEEE Transactions on Geoscience and Remote Sensing, 47(7), 1999-2013, doi:10.1109/TGRS.2008.2011617
- Naeimi, V., C. Paulik, A. Bartsch, W. Wagner, R. Kidd, S.-E. Park, K. Eiger, and J. Boike, 2012, ASCAT surface state flag (SSF): Extracting information on surface freeze/thaw conditions from backscatter data using an empirical theshold-analysis algorithm. IEEE Transactions on Geoscience and Remote Sensing, 50(7), 2566-2582, doi:10.1109/TGRS.2011.2177667
Websites:
- Advanced SCATterometer (ASCAT) | EUMETSAT Website, https://www.eumetsat.int/ascat
- Metop Series | EUMETSAT Website, https://www.eumetsat.int/our-satellites/metop-series
- EUMETSAT, Home Page - H SAF Official web site, http://hsaf.meteoam.it/
Data citation
Please cite the data as follows:
Daily global maps:
Globally gridded daily 5-day running mean / composite ASCAT soil moisture maps (https://doi.org/10.25592/uhhfdm.10467, https://doi.org/10.25592/uhhfdm.13101) derived at the Integrated Climate Data Center (ICDC) from H SAF (2022): ASCAT Surface Soil Moisture Climate Data Record v7 and v7 Extension 12.5 km sampling - Metop, EUMETSAT SAF on Support to Operational Hydrology and Water Management, https://doi.org/10.15770/EUM_SAF_H_0009 , last access: July 4 2023.
Monthly global maps:
Globally gridded monthly mean ASCAT soil moisture maps (https://doi.org/10.25592/uhhfdm.10468, https://doi.org/10.25592/uhhfdm.13103) derived at the Integrated Climate Data Center (ICDC) from globally gridded daily 5-day running mean / composite soil moisture maps (https://doi.org/10.25592/uhhfdm.10467, https://doi.org/10.25592/uhhfdm.13101).
In addition you need to cite:
- Wagner, W., G. Lemoine, and H. Rott (1999), A method for estimating soil moisture from ERS scatterometer and soil data. Remote Sensing of Environment, 70(2), 191-207, doi:10.1016/S0034-4257(99)00036-X
- Naeimi, V., K. Scipal, Z. Bartalis, S. Hasenauer, and W. Wagner, 2009, An improved soil moisture retrieval algorithm for ERS and MetOp scatterometer observations, IEEE Transactions on Geoscience and Remote Sensing, 47(7), 1999-2013, doi:10.1109/TGRS.2008.2011617
- Naeimi, V., C. Paulik, A. Bartsch, W. Wagner, R. Kidd, S.-E. Park, K. Eiger, and J. Boike, 2012, ASCAT surface state flag (SSF): Extracting information on surface freeze/thaw conditions from backscatter data using an empirical theshold-analysis algorithm. IEEE Transactions on Geoscience and Remote Sensing, 50(7), 2566-2582, doi:10.1109/TGRS.2011.2177667
Because the data are based on measurements of the EUMETSAT METOP-ASCAT sensor EUMETSAT has to be mentioned:
- All H-SAF products are owned by EUMETSAT, and the EUMETSAT SAF Data Policy applies. They are available for all users free of charge.
Users should recognise the respective roles of EUMETSAT, the H-SAF Leading Entity and the H-SAF consortium when publishing results that are based on H-SAF products. EUMETSAT's ownership and intellectual property rights into the SAF data and products is best safeguarded by simply displaying the words "(C) EUMETSAT" under each of the SAF data and procuts shown in a publication of website.