Award Winners 2024
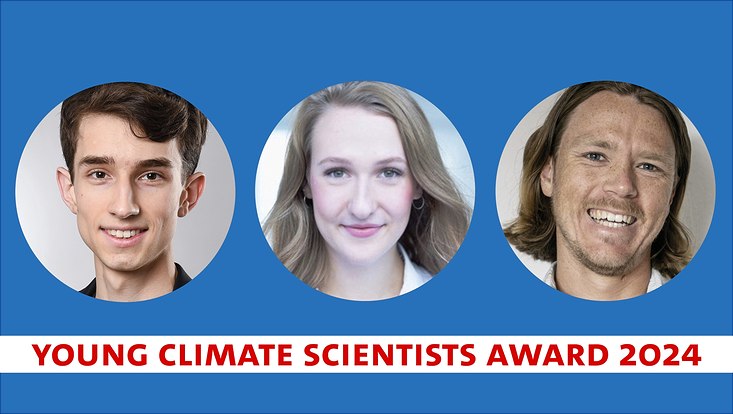
Thomas Wasilewski/UHH/CEN
“Each year, hundreds of papers are submitted and reviewed at the University of Hamburg – and there are definitely some gems in the mix,” says Prof. Kay-Christian Emeis, a jury member and presenter of the Young Climate Scientists Award 2024. This year, 27 top-notch papers were submitted for consideration. The three best papers were recently presented at a ceremony with representatives of the Gesellschaft Harmonie von 1789 e.V., which finances the award: first place went to David Hafezi Rachti for his bachelor’s thesis in Meteorology (€ 5.000); second place went to Cathrin-Charlotte Basche for her bachelor’s thesis in Geography (€ 3.000), and third place went to Kai Bellinghausen for his master’s thesis in Ocean and Climate Physics (€ 2.000). In the jury’s view, thanks to their courage and creativity, the winners successfully broke new ground and overcame the boundaries between disciplines.
How the atmosphere affects carbon uptake in forests
David Hafezi Rachti applied a new, innovative machine learning technique to identify interactions in the carbon cycle. He focused on forests, which serve as key sinks in the global carbon cycle and regulate the speed of climate change. How efficiently ecosystems can absorb carbon is subject to substantial fluctuations shaped by the biosphere, lithosphere and atmosphere. In addition, stress factors like extended dry periods are relevant factors and time-delayed ecosystem responses also have to be taken into account. In his paper, Rachti combines findings from machine learning, a grasp of key biological processes, and theory on atmospheric exchange processes. He shows how machine learning offers new opportunities for addressing complex, perennial problems: he adapts machine learning techniques to investigate the role of meteorological variability in connection with the carbon budgets of forest ecosystems and analyzes a total of 15 deciduous forests. His conclusion: available water is the most important factor for fluctuations in the carbon budget.
How climate change is shrinking glaciers in the Himalayas
Cathrin-Charlotte Basche investigated the dwindling of glaciers in the Central Himalayas in response to climate change. To do so, she took a closer look at changes in Tibet’s Paiku Co Basin from 1988 to 2021 and identified the connection to global warming. In this regard, she analyzed satellite-based and regional climate data and their interrelations. Her work fills an important gap, as there are virtually no glaciological works focusing on the Central Himalayas region. In her paper, she shows that there have been changes in all four glaciers surveyed: the glacier tongues have retreated markedly, forming glacial lakes at their ends. Her findings are directly relevant for the water supply for the people living in the region, and for the local environment. Said findings could be applied to supra-regional reconstructions of glacier dynamics in the Himalayas, while also offering methodologically innovative approaches transferrable to other contexts.
Predicting storm surges on the Baltic days in advance
Kai Bellinghausen presented one of the first applications of machine learning for short-term oceanographic forecasts for the Baltic. He developed an algorithm for predicting daily extreme water levels along the Baltic coast, integrating atmospheric influences like wind, temperature and precipitation to do so. In this regard, the goal was to approach the Baltic as a complex system: here, the main drivers of storm surges are the effects of pressure and wind, as well as a condition known as “prefilling” – an increase in the Baltic’s volume, produced by the influx of water masses from the North Sea via the Danish straits. Depending on the coastline’s orientation, the patterns of storm-surge drivers change. In his paper, Bellinghausen successfully applies an interdisciplinary approach that combines a firm grasp of storm-surge processes with the world of machine learning. In addition to processing extensive datasets from meteorological reanalysis and sea-level observations, he systematically tests various configurations of the machine learning model (Random Forests) and employs a range of methods to assess the model’s statistical predictive quality. Given the serious damage that storm surges can produce, the forecasting system is highly relevant for the populaces of countries bordering the Baltic and can, given its short-term nature, be used as a prototype for more complex forecasting systems.